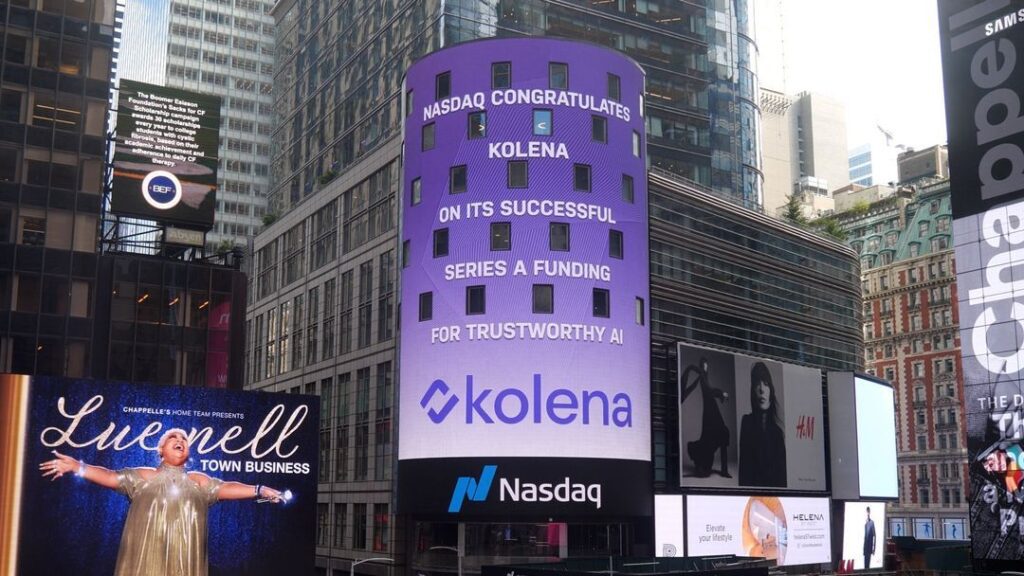
Marc Andreesen recently wrote that AI will save the world. Only time will tell whether he’s right, but there’s one thing we already know for sure: AI can’t save the world until we can trust it.
We hear almost daily about the real-world impacts of AI that isn’t fully trustworthy. Hallucinations creating cybersecurity risks within companies’ own tech stacks or exposing valuable data. Lawsuits alleging mistaken arrests due to biased AI facial recognition systems. Self-driving cars failing to recognize pedestrians or emergency vehicles.
These impacts are also being felt within organizations that are commercializing AI products. When the product or sales team makes promises that the engineering team’s AI model can’t keep, customer trust is lost and the company’s own cohesion is jeopardized.
So how can we plot a path toward AI that we as a society can rely upon?
In simple terms, we need to invert the old Cold War maxim: We need to verify before we can trust.
The Current State of Trust in AI
AI today stands where software was decades ago: a tool whose potential has yet to be fully understood, and which needs to prove itself to the communities of builders, buyers and regulators it impacts.
Just as no single person or group was responsible for getting software over the threshold of trust to achieve its present ubiquity, it will take a worldwide movement toward AI testing and quality to create trustworthy AI.
This movement is already underway. Before we get to that, though, let’s examine the problem from the perspective of each of the communities above.
Builders – the teams and organizations that are creating AI and AI-enabled products – are rapidly converging around an ‘AI-as-a-Service’ approach, using massive foundation models to handle basic, common AI functions and then building specialized capabilities on top of them.
Unfortunately, this approach immediately creates conditions that can jeopardize trust within the organization. Due to the lack of effective testing tools, the specialized team has limited visibility into the ‘guts’ of the foundation model, making their product vulnerable to potentially serious unexpected behavior. The builders can’t effectively test the model, so they can’t trust it – and their peers in the enterprise can’t trust them to deliver a reliable product.
Many buyers are still AI skeptics. They’ve heard the hype, but they know that purchasing the wrong product or system can cost them their jobs.
What’s worse, the current state of AI / ML model testing is almost tailor-made to instill a false sense of security in them. A buyer evaluating a model with a 99% aggregate accuracy score might assume it will be reliable enough for their needs – without realizing that the very task they need the model to handle most could be part of that nebulous 1%.
When a model touted as ‘99% accurate’ fails in a crucial scenario, many buyers not only lose trust in the builders, but in AI as a whole.
Regulators are currently in a no-win situation with AI, lacking the testing and validation frameworks to create solid quality assurance processes before models are released into the wild. Data governance and model monitoring techniques are helpful for evaluating models post-deployment, but without further innovation in pre-deployment AI testing and quality assurance, the regulatory puzzle is missing its most important piece.
Imagine if the FDA did away with clinical trials in favor of monitoring new drugs after they had already been prescribed. That’s essentially where we stand with regulation for AI / ML models today.
The Revolution Has Already Begun
So where do we go from here? Back to our software analogy, to start. Testing practices for software once resembled today’s practices for testing AI / ML models: They were manual, tedious, over-reliant on experimentation and enormously time-consuming, resulting in slower development cycles and unreasonable costs for high quality software solutions.
Luckily, the global software community decided to find a better way by implementing testing frameworks like unit testing, regression testing and others. They came together and developed systems, frameworks, tools and best practices to create higher quality products.
Today, almost all software testing is automated and consistently delivers high-quality results at vastly lower costs. Not coincidentally, software has also become a trusted part of our lives.
The global movement to do the same for AI model testing and quality has already begun, and it will only continue to grow. AI teams and engineers know we need to find better solutions for validating AI models in order to ensure a trustworthy AI-enabled future.
Kolena is helping to drive this movement. We’re building AI / ML model testing and validation solutions that help developers build safe, reliable, and fair AI systems by allowing companies to instantly create razor-sharp test cases from their data sets, enabling them to scrutinize models in the precise scenarios those models will be entrusted to handle in the real world.
In addition, Kolena is bringing successful software concepts like unit testing and regression analysis into the AI development framework, transforming AI / ML testing from experimentation-based into an engineering discipline that can be trusted and automated.
Our recent $15 million Series A funding round led by Lobby Capital will help us further advance the AI testing revolution by building out our solutions, expanding our research efforts, and collaborating with ML communities and regulators to build unified frameworks for AI testing and validation while establishing key metrics and best practices.
Kolena is deeply grateful to everyone that makes up the vibrant and supportive AI / ML community – especially our customers, who are amazing sources of inspiration and motivation.
If you are an AI leader tasked with adopting AI in your organization, or a policy maker working on AI safety and standardization, please reach out and get in touch. We would love to connect and collaborate.
All of us have a critical role to play in building the path to trustworthy AI.
Sincerely,
Mohamed Elgendy, CEO and Co-Founder, Kolena
PS. I’ll be writing monthly articles sharing notes and lessons learned on how to build trustworthy AI. Subscribe below to follow our news.
Mohamed Elgendy is the CEO and co-founder of Kolena, a startup that provides robust, granular AI / ML model testing for computer vision, generative AI, natural language processing, LLMs, and multi-modal models, with the goal of ensuring trustworthiness and reliability in an increasingly AI-driven world.